In order to understand an intrusion chain sometimes it is necessary to deal with a a lot of information from different sources at the same time. This can be really a challenge process.
One of the key points to success is to create a proper timeline of all the events. The timeline of events can be reviewed manually; in some cases using tools like Microsoft Excel, but in some cases tools like Splunk might make our life easier.
One of the key points to success is to create a proper timeline of all the events. The timeline of events can be reviewed manually; in some cases using tools like Microsoft Excel, but in some cases tools like Splunk might make our life easier.
Using the same Gozi malware I wrote about about some days ago, which it is being really very active at the moment, I am going to explain the process to create a proper timeline for evidence from an infected system (files, registers, logs, artifacts..), the memory dump of that same system, and the network traffic capture generated by that system. Then, one the timeline has been created, I will import that data into Splunk, which allows to perform advance searches or even create your own correlation rules base on the data gathered
Supertimeline with Palso: log2timeline.py and psort.py
A very good document of what is supertimeline and the tools involved, is in this post FORENSICS EVIDENCE PROCESSING – SUPER TIMELINE from my friend Luis Rocha. In that post Luis explains how to gather the image of a compromised system to create the timeline.
Log2timeline.py is the main tool in charge of extracting the data. It has lot of parsers.
In the case of my analysis I am interesting in the parsers for PCAP files and Volatility memory dump.
In the case of my analysis I am interesting in the parsers for PCAP files and Volatility memory dump.
Timeline from the files system in a Virtual Enviroment
The first step is to extract the evidence from the filesystem. In this case, as am I dealing with a Virtual Machine I can straight forward mount the file where the OS resides. This can be done with the command vmdkmount in a MacOSX system running Vmware Fusion. But similar approach is done in any Virtual environment with ESX or similar.
vmdkmount "Virtual Disk-000001.vmdk" /mnt/vmdk/
After that, it is necessary to point log2timeline.py to the directory where the files are mounted. The first parameter is the file where to store the output of the analysis (plaso.dump). This will start processing all the data. After a few hours of processing, there are more than 7M events processed and the output file 'plaso.dump' is around 500MB of size.
$ log2timeline.py /mnt/hgfs/angel/malware/gozi/analysis3/plaso.dump /mnt/vmdk/vmdk2 The following partitions were found: Identifier Offset (in bytes) Size (in bytes) p1 1048576 (0x00100000) 100.0MiB / 104.9MB (104857600 B) p2 105906176 (0x06500000) 99.9GiB / 107.3GB (107267227648 B) Please specify the identifier of the partition that should be processed: Note that you can abort with Ctrl^C. p2 The following Volume Shadow Snapshots (VSS) were found: Identifier VSS store identifier Creation Time vss1 581c4158-cbea-11e5-8f4b-000c296cf54c 2016-02-10T08:15:27.834308+00:00 vss2 581c41e0-cbea-11e5-8f4b-000c296cf54c 2016-02-10T15:55:13.094999+00:00 vss3 17d17138-d1c0-11e5-8574-000c296cf54c 2016-02-12T19:41:28.401546+00:00 vss4 73502ebb-d1c1-11e5-83be-000c296cf54c 2016-02-12T20:02:28.619844+00:00 vss5 b61505ac-d1c7-11e5-8ecf-000c296cf54c 2016-02-22T16:51:50.095472+00:00 vss6 b6150618-d1c7-11e5-8ecf-000c296cf54c 2016-02-23T02:00:13.750478+00:00 vss7 b61506a9-d1c7-11e5-8ecf-000c296cf54c 2016-02-23T09:38:15.072461+00:00 Please specify the identifier(s) of the VSS that should be processed: Note that a range of stores can be defined as: 3..5. Multiple stores can be defined as: 1,3,5 (a list of comma separated values). Ranges and lists can also be combined as: 1,3..5. The first store is 1. If no stores are specified none will be processed. You can abort with Ctrl^C. 1,7 Source path : /mnt/vmdk/vmdk2 Is storage media image or device : True Partition offset : 105906176 (0x06500000) VSS stores : [1, 2, 3, 4, 5, 6, 7] 2016-02-23 12:21:09,283 [INFO] (MainProcess) PID:33208 <frontend> Starting extraction in multi process mode. 2016-02-23 12:21:16,271 [INFO] (MainProcess) PID:33208 <interface> [PreProcess] Set attribute: sysregistry to /Windows/System32/config
...
...
...
2016-02-23 17:53:08,130 [INFO] (MainProcess) PID:3421 <multi_process> Extraction workers stopped.
2016-02-23 17:53:10,363 [INFO] (StorageProcess) PID:3430 <storage> [Storage] Closing the storage, number of events processed: 7191131
2016-02-23 17:53:10,373 [INFO] (MainProcess) PID:3421 <multi_process> Storage writer stopped.
2016-02-23 17:53:10,391 [INFO] (MainProcess) PID:3421 <log2timeline> Processing completed.
Timeline from a memory dump
Again, as I am dealing with a Virtual Machine, I can use the files where the memory has been dumped from the Virtual System to generate the file memory dump for Volatility.
In MacOSX this is done with the application 'vmss2-core-mac64', which can be dowloaded from VMWare website.
vmss2core-mac64 -W "Windows 7 x64-11a96bd1.vmss" "Windows 7 x64-11a96bd1.vmem"
- W: this is to get the memory in Windebug format, which can be easily read by volatility
-*.vmss is the file containing the information of memory of the Virtual Machine
-*.vmem is the file which contains the memory dump
Once this is done, it is time to extract the timeline of the memory with Volatility through the plugin "timeliner". The output is stored in a temporal file "timelines.body"
Last step is to parse the data generated by Volatility to the 'mactime' format and include in the original file, where all the timeline has been stored so far (plaso.dump).
To do so, I run the following command:
-*.vmss is the file containing the information of memory of the Virtual Machine
-*.vmem is the file which contains the memory dump
Once this is done, it is time to extract the timeline of the memory with Volatility through the plugin "timeliner". The output is stored in a temporal file "timelines.body"
$ volatility --profile=Win7SP1x64 -f memory.dmp --output=body timeliner > timelines.body
Last step is to parse the data generated by Volatility to the 'mactime' format and include in the original file, where all the timeline has been stored so far (plaso.dump).
To do so, I run the following command:
$ log2timeline.py --parser "mactime" plaso.dump timelines.body
Timeline from network capture
For this step I use the parser 'pcap' against the capture file. The output will be stored in the 'plaso.dump' file as well.
$ log2timeline.py --parser "pcap" plaso.dump capture.pcap
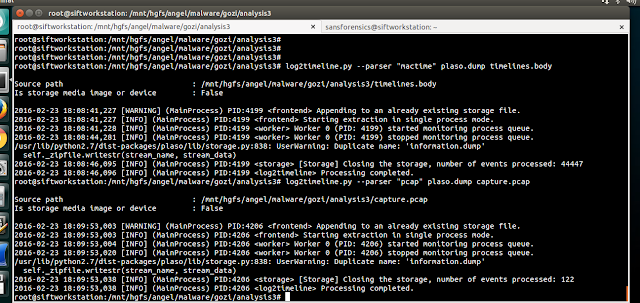
Generating the CSV with the sorted timeline
plaso.dump contains already all the timeline data from the network, the memory and the system. Now it is time to sort the data and generated a CSV file which can be easily open with excel or any other tool. This is done with the command psort.py.
But our plaso.dump is a 600MB file, full of data, so it is better to only extract all the data which I think is relevant for the analysis of this incident.
In this case, as filter, I choose all the data from the day before the incident happened. This is done with the 'date'
$ psort.py -o L2tcsv plaso.dump "date > '2016-02-22 23:59:59'" >supertimeline.csv
....
*********************************** Counter ************************************
[INFO] Stored Events : 7235700
[INFO] Filter By Date : 7208053
[INFO] Events Included : 27647
[INFO] Duplicate Removals : 5168
In the end my supertimeline contains around 27k rows.
Analysing the data with Excel
When importing the 'supertimeline.csv', and after creating filters, I can see that there are events coming from the system, but also from the pcap file (capture.cap) and the memory timeline (timelines.body), as expected. This means that the timeline is properly consolidate with all the data from different sources.
Looking at the possible filter in one of the rows I see diversity of captured events. Since event logs, many different Windows artifacts, register, MACB time, capture traffic, etc.
This gives an idea of the amount of data extracted.
This gives an idea of the amount of data extracted.
However, if I have to deal with a CSV file much bigger that this, which is something quite command, or I am dealing with and incident involving many hosts hence I have several different CSV/Timelines the XLS approach could be a nightmare and lot of manual work.
This is when tools like Splunk show up in the game :)
This is when tools like Splunk show up in the game :)
Analysing the data with Splunk
To import the CSV file in Splunk is really straight forward process. From the search menu, there is already an option to add data.
While importing I already can see the data is there.
The only recommendation is to create a unique index for the specific investigation, in case the Splunk instance is not only used for this purposed. Also, I recommend to insert the real name of the host in the hostname, so it is possible to perform searches base on a specific host (in case there are multiple timelines from multiple hosts), which can facilitate the correlation through different host, for example to detect a possible lateral pivoting.
In the search, we can already start making searches for our specific target host "Windows7".
I could identified all the DNS responses with a simple query
In next post I will go deeper with the Splunk analysis and queries in order to understand what has happened